일 | 월 | 화 | 수 | 목 | 금 | 토 |
---|---|---|---|---|---|---|
1 | 2 | 3 | 4 | 5 | 6 | 7 |
8 | 9 | 10 | 11 | 12 | 13 | 14 |
15 | 16 | 17 | 18 | 19 | 20 | 21 |
22 | 23 | 24 | 25 | 26 | 27 | 28 |
29 | 30 |
- 상관관계
- correlation
- Retry
- chatGPT
- login crawling
- spark udf
- session 유지
- GenericGBQException
- Counterfactual Explanations
- BigQuery
- airflow subdag
- API Gateway
- integrated gradient
- gather_nd
- XAI
- 공분산
- UDF
- 유튜브 API
- TensorFlow
- API
- youtube data
- flask
- hadoop
- Airflow
- top_k
- grad-cam
- GCP
- requests
- subdag
- tensorflow text
- Today
- Total
데이터과학 삼학년
Heterogeneous Graph Neural Networks for Extractive Document Summarization (2020) 본문
Heterogeneous Graph Neural Networks for Extractive Document Summarization (2020)
Dan-k 2022. 5. 6. 19:01Heterogeneous Graph Neural Networks for Extractive Document Summarization
Abstract
- cross-sentence realtion를 학습하는 것이 document summarization을 추출하는 주요 방법
- 그중 직관적인 방법은 graph based neural network에 넣어 inter sentence의 관계를 찾아내는 것임
- 논문에서 제안한 모델은 크게 graph initializer, heterogeneous graph layer, sentence selector 로 구성된 모델
- single document, multi document summarization이 모두 가능한 모델로, 공개 데이터셋을 가지고 bert를 제외한 타 모델과 비교했을때 비교적 좋은 성능(Rouge)을 가지는 것으로 확인
1 Introduction
- original 문장을 재구성하여, summary를 만드는 연구들은 최근 딥러닝 적용으로 성공적으로 보임
- 주로 사용한 딥러닝 모델은 encoder-decoder framework를 이용하는 것으로, 각 문장들을 NN을 이용해 encode 시킴
- document에서 주요 summary를 뽑는 핵심 단계는 cross-sentence 관계를 모델링하는 것임
- 대부분 현존하는 모델은 RNN기반으로 cross-sentence관계를 모델링함
- RNN기반의 모델은 이미 알려진대로 긴 문장 수준에서는 제대로 작동하지 않음
- 더 직관적인 방법은 sentence간 관계를 graph structure로 나타내는 것인데, 상당히 도전적인 일임
- 전통적인 방법은 inter-sentence간의 cosine similarty를 이용해서 연결관계를 만드는 것이고, 대표적인 예가 LexRank, TextRank임
- 최근 연구들은 Approximate Discourse Graph (ADG), Rhetorical Structure Theory (RST) graph이런 것들인데, propagation error 문제, 외부 툴에 의존적이라는 문제가 있음
- transformer를 이용해 문장간 pairwise interaction을 학습시키는 연구들도 이루어지고 있음
- 그러나 여전히 효율적인 graph structure를 구성하여 summary를 뽑아내는 것은 연구과제임
- 이 연구에서 sentence level의 nodes를 추가하는 것 뿐만 아니라, semantic units도 추가하였고, 이를 통해 문장간 연결성을 극대화함
- 좀 더 진보적인 feature로 entities나 topics를 사용할 수 있었지만, 이 paper에서는 간단하게 words를 사용했다 (semantic units으로)
- 각 문장들은 포함된 words로 서로 연결됨
3 Methodology
- document안에 있는 n개의 sentence를 이용해서 extractive summarization 추출 가능
- extractive summarization은 해당문서내에서 원본 글자 그대로 중요한 문장을 추출하여 요약하는 방법
- 문장들을 이용해 graph layer를 만들고 sentence selector가 1,0인 label를 예측하도록 모델을 학습 시킴
- 즉, summarization으로 이용할 문장들을 뽑아내는 모델이라고 생각하면 됨
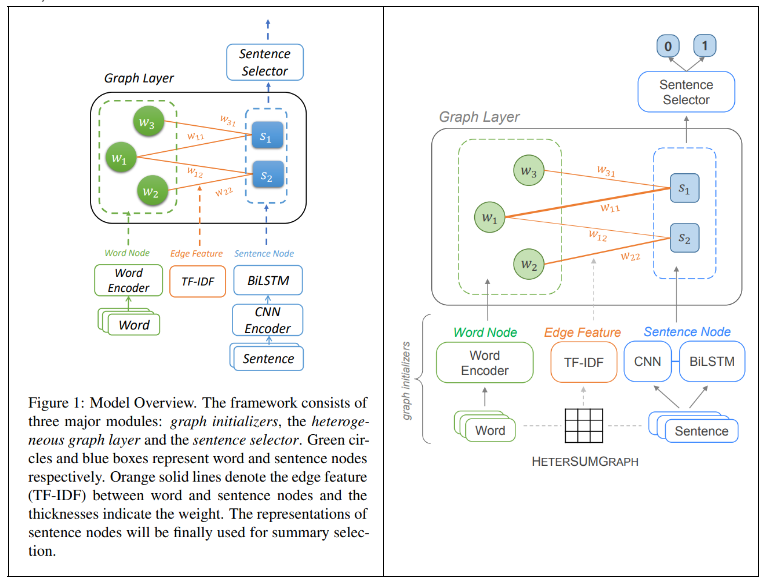
3.1 Document as a Heterogeneous Graph
- 모델은 크게 3가지로 구분되어짐
- graph initializer
- node와 edge를 만들고, encode
- heterogeneous graph layer
- node representations를 업데이트함
- Graph Attention Network를 이용해 word와 sentence node사이의 messages를 passing하면서 학습
- sentence selector
- summaries를 위한 labels를 예측하는 모델로 sentece를 선택해냄
3.2 Graph Initializers
- Word Node
- word embedding한 값 사용 → pre-trained model인 GloVe를 사용하여 구성
- Sentence Node
- CNN을 이용하여 local n-gram feature 추출 → lj
- BiLSTM을 이용하여 sentence embedding 을 구함 → gj
- lj 와 gj를 concatenation한 Xsj = [lj ; gj ] 구성
- Edge Feature
- word-sentence 관계를 tf-idf를 사용하여 weighted edge로 나타냄
3.3 Heterogeneous Graph Layer
- G = {V, E}
- word-word, sentence-sentence간 연결은 고려하지 않음
- Graph Attention Network(GAT)를 이용해 각 vertex 연결된 edge weight를 학습시킴
- gradient vanishing 문제를 해결하기위해 일정 iteration마다 residual connectin을 적용
3.4 Sentence Selector
- heterogeneous graph로부터 summary를 만들어내고, summary구성을 위해 포함된 sentence nodes를 추출할 필요가 있음
- node classification : cross-entropy loss를 사용하여 학습
- Trigram blocking : sentence를 score 순서로 내림차순 한 이후 겹치는 trigram이 있는 문장을 제거하는 과정
1 : The quick brown fox jumps over the lazy dog.
2: The quick white fox jumps over the slow dog
3.5 Multi-document Summarization
- multi-document summarization을 실행하기 위해 document 간의 관계를 이해하는것이 중요
- 여러개의 문서에서 한개의 summarization을 추출하는 것이 multi-document summary
- single-document summary 는 한개의 document에서 한개의 summary를 추출하는 것
- 문서간 관계를 정립하기위해, 각 문서의 supernodes를 추가하여 반영함
- 이를 통해 쉽게 single -> multi document summarization으로 확장 가능
4 Experiment
- 활용 데이터 셋
- single : CNN/DailyMail NYT50
- multi : Multi-News
- 비교 모델
- Ext-BiLSTM
- Ext-Transformer
- HETERSUMGRAPH
- 평가방법 : Rouge metrics
- 모델로부터 추출된 summary가 ground truth인 summary와 비교
- summary를 n-gram으로 word set을 만들어, 모델이 예측한 summary set의 갯수 중 실제 summary 값과 얼마나 겹치는지 나타내는 용도
5 Results and Analysis
- 컴퓨팅 문제로 이 논문에서는 bert를 안썼기때문에 bert를 쓰지않은 모델들과 비교함
- single document 비교결과
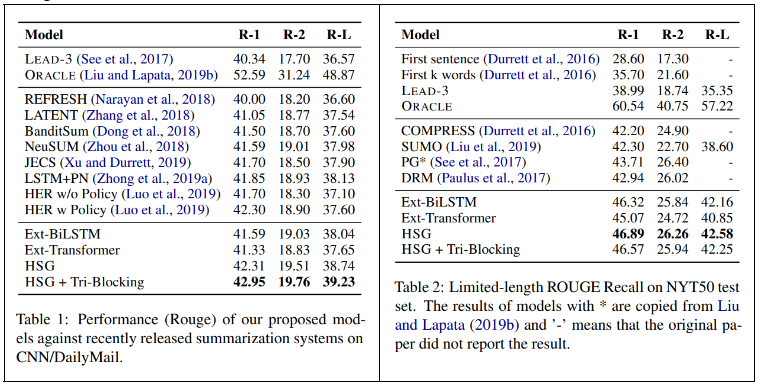
- 이 논문에서 적용한 필터조건을 CNN/DailyMail 데이터셋에 동시적용하여, 다시 모델 비교한 결과
- multi document 비교결과
6 Conclusion
- 논문에서 제안한 모델은 문장간 복잡한 관계를 더 잘 빌드할 수 있다는 장점이 있고, single → multi document에 대한 summarization도 가능
- bert를 사용하지 않은 모델들과 비교했을때 공개데이터셋에서 좋은 성능을 가지는 것으로 확인
Reference
'Papers' 카테고리의 다른 글
RECSIM: A Configurable Simulation Platform for Recommender System (2019) (0) | 2021.06.02 |
---|---|
Multivariate recurrence plots (2004) (0) | 2021.01.04 |
Forecasting at Scale (2018) - Prophet 소개 (0) | 2020.12.10 |
Anomaly Detection in Time Series Data Based on Unthresholded Recurrence Plots (2018) (0) | 2020.12.02 |
Universal Sentence Encoder (2018) (0) | 2020.08.06 |